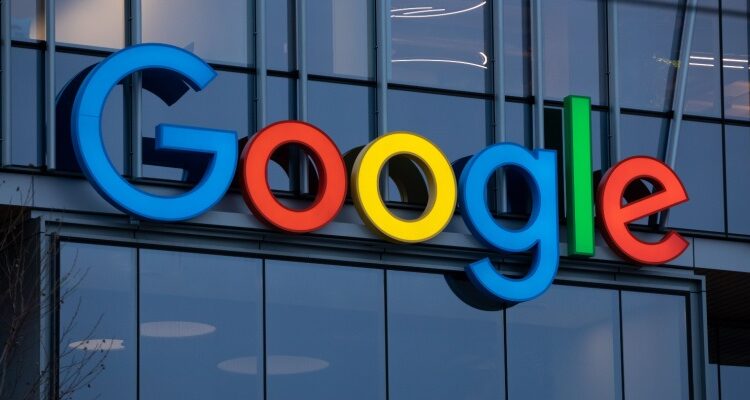
Google’s advanced generative AI models, Gemini 1.5 Pro and 1.5 Flash, have been marketed for their capability to process and analyze massive amounts of data.
New studies, however, are challenging these promises, suggesting that these models may not perform as effectively as claimed.
- New studies question the efficiency of Google’s AI models, Gemini 1.5 Pro and 1.5 Flash, in handling large data.
- The models show a 50% accuracy rate in reasoning over video content.
- These findings add to the growing skepticism about generative AI technology’s potential and limitations.
Google’s AI Models Questioned: Studies Highlight Data Handling Issues
Handling Large-scale Data: A Challenge?
Recent research studies indicate that Google’s Gemini models may struggle when dealing with large datasets. Contrary to Google’s claims that these models can analyze data equivalent to the length of “War and Peace,” the models were found to give correct answers to questions only 40% to 50% of the time.
Marzena Karpinska, a co-author of one of these studies and a postdoc at UMass Amherst, shared her observation. She stated that although Gemini 1.5 Pro technically can process long contexts, it often fails to genuinely comprehend the content.
Limitations of Context Window
A model’s ‘context window’ refers to the input data it uses to generate output. Google’s latest Gemini models can handle up to 2 million tokens as context, equivalent to about 1.4 million words, two hours of video, or 22 hours of audio. This surpasses any other commercially available model.
Despite this, the studies found that these models struggled to verify claims that required comprehensive consideration of large documents. They also found it challenging to interpret implicit information, which might be clear to human readers but isn’t explicitly stated in the text.
Struggling with Video Analysis
In a separate study, the ability of Gemini 1.5 Flash to reason over videos – that is, sift through and answer questions about their content – was examined.
The model’s performance was less than stellar in this task. It transcribed handwritten digits from a series of images accurately only about 50% of the time, and this accuracy rate dropped further when more digits were involved.
Is Google Overcommitting?
While these studies have not yet been peer-reviewed and did not test the latest Gemini models, they suggest a possibility of Google overcommitting in its claims. Other models, like OpenAI and Anthropic, also didn’t perform well in the tests.
However, the context window has been a focal point in Google’s marketing strategy, setting it apart from its competitors.
Generative AI technology is facing increased scrutiny as businesses and investors express frustrations with its limitations.
According to Boston Consulting Group surveys, around half of the polled C-suite executives are skeptical about substantial productivity gains from generative AI. Moreover, they expressed concerns about potential errors and data compromises.
The Need for Improved Benchmarks
Marzena Karpinska and Michael Saxon, a PhD student at UC Santa Barbara and co-author of the second study, propose the need for better benchmarks and increased emphasis on third-party critiques. They caution the public to take grandiose claims about generative AI with a grain of salt, especially when companies do not share detailed information about long-context processing.
Join our newsletter community and get the latest AI and Tech updates before it’s too late!
Leave a Reply